Have AI Brain to Image Systems Arrived? Not quite.
Our thoughts have always been the starting point for our creations - from building machines to writing stories. But what if our thoughts could become tangible before our very eyes? This notion - once far-fetched - has started to feel more within reach due to the advent of text to image AI image generation.
On Friday, March 3rd a white paper started to go viral on Twitter that some thought might signal the advent of thought to image was upon us, the wonder, awe and fear ensued as people pondered the notion of our minds being read and visualized by AI.

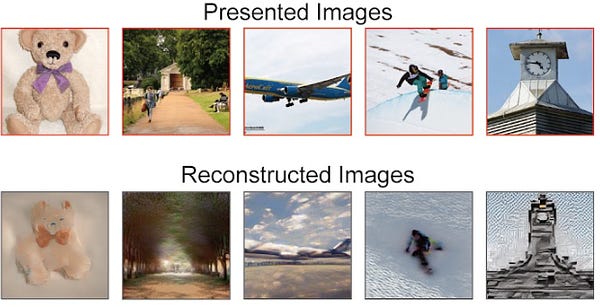
While the technology to create images directly from brain activity has proven possible in recent decades - with this being the latest research on the subject - it isnโt a thought to image system as some hoped, and others feared.


To create a machine that can draw what you're thinking of, three steps are needed.
Step One is a matter of how your brain works, since the brain activity of someone imagining something is different from the brain activity in the visual cortex of someone actually seeing something, and there must be some correlation between the two types of brain activities that can be picked out by a functional magnetic resonance imaging (fMRI) scan. Step Two requires mapping brain activity seen in an fMRI scan to an image or video representation, with a perceptible difference between brain activity for different objects. There needs to be a consistent and perceptible difference in the brain activity of someone looking at an elephant versus someone looking at a car. Step 3 is the ability to reconstruct or draw images from the encoded representation obtained in Step Two.
By perfecting these three steps, we may be able to generate tangible images from our thoughts in the future.
Very recent advances with generative AI, particularly with diffusion models, are able to do Step Three very well now. For this reason, the paper "High-resolution image reconstruction with latent diffusion models from human brain activity" by Yu Takagi and Shinji Nishimoto, released in December of 2022, has generated a lot of online fascination at how vivid the images an AI had drawn directly from brain activity were.
What Stable Diffusion and other rapidly improving image-generation AI models provide is a new way to interpret this different brain activity and actually visualize them. Previous approaches, before we had the computationally cheap Stable Diffusion, produced fuzzy forms and images that did not look meaningful, such as these images from "Deep image reconstruction from human brain activity" in 2019:
This is a powerful example of how our new, open-source AI models can synergistically combine with older technologies to produce astonishing results. There has been no significant change in fMRI technology for reading brain activity, itโs just that we have much better tools at mapping this activity into latent representations that can be turned into meaningful images through Stable Diffusion.
Itโs easy to forget how recent and advanced the 2019 papers using self-supervised and GAN models already were. Back in 2011, a team in UC Berkeley created some of the earliest reconstructions of video clips through brain activity:
This was before generative AI, so in their approach, they actually used a statistical maximum likelihood method. They found all the video clips that created the most similar response to brain activity that was detected, and created an โaverage clipโ across those similar video clips. The resulting videos could reconstruct a lot of the forms and colors, but were of course a lot less compelling than generated images from diffusion models. Now, generative AI has removed the need for using such crude methods.
So we have reasonable proofs of concepts in Step 2 and Step 3, but how about Step 1? Can we read the brain activity of imagined scenes and images, and not just things people are directly looking at with their own eyes?
This depends on the individual. According to the paper โThe neural correlates of visual imagery vividnessโAn fMRI study and literature reviewโ in published 2018, visual imagery vividness is a subjective experience that varies across individuals and depends on several factors, such as attention, memory, emotion, motivation, and cognitive style. So some individuals activate similar visual cortex activity as has been used for the brain-reading proofs of concepts when theyโre just imagining they see things, but others do not. This makes sense on a fundamental level, since people often overestimate how much they are able to visualize, and some people have aphantasia, or are unable to visualize at all.ย
Our brains are very good at tricking us into thinking that we are fully visualizing something even when we are not. Imagine a bicycle, picture it clearly in your head. Is the image clear? Now draw a bicycle out on paper. It is likely that you find that you canโt really remember where the struts and supports and chains of a bicycle actually go, despite tricking yourself into thinking you had a clear image of a bicycle just moments before. The brain is very good at filling the gaps of what we canโt visualize by drawing our attention away from it. Luckily, models like Stable Diffusion and other generative models are also very good at filling in gaps and in-painting.
Some day, they may perform in-painting on behalf of images generated from reading our minds. Allowing for a kind of camera for imagination, doing with neurones what cameras do with photons - the advent of nuerography isnโt here yet - but itโs hard to image it isnโt inevitable.